NYTimes v. OpenAI: A Death Sentence Foretold
Unless they can arbitrage their way around the Constitution, generative algorithms will become a financial black hole for investors.
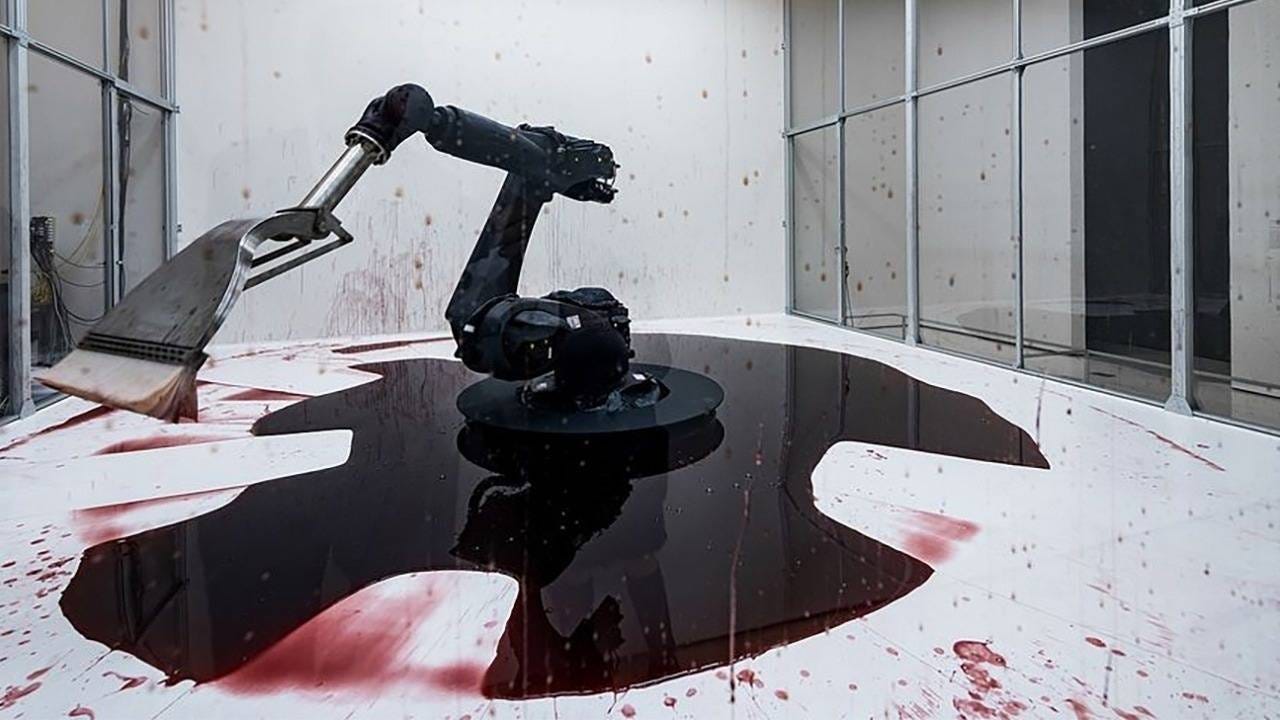
Probably my favorite concept in economics, and one fundamental to the mission of this publication, is that of the “externality.” An externality is a cost (or sometimes benefit) not borne by the actual purchaser of a good or service. One classic negative externality is the pollution generated by a car, which harms everyone, rather than just the person who benefits from driving. A classic positive externality is education, where the benefits of an educated society spread far beyond whoever “pays” for the schooling.
Externalities are, in short, places where the market fails to produce optimal resource allocation. They are places where symbolic math breaks down in the face of the complexity of flesh and blood. They require social and legal intervention, because left to run unfettered, they produce negative, in some cases catastrophic, outcomes. A huge portion of the most intractable modern political conflicts are based on one side’s ideological refusal to truly reckon with the existence of externalities in a “free” market.
Yesterday the New York Times sued OpenAI and Microsoft for, in economic terms, benefitting from the positive externalities created by good journalism. This is also sometimes referred to as a “free rider” problem – the Times is effectively accusing OpenAI of being a parasite, taking its reporters’ work for free, then commercializing the results in a way that competes with and threatens to crowd out the original product.
Legal experts are already saying it could be a landmark case. You can read the full text of the complaint here. I have only had a chance to get an overview of the 69 pages, but there will be plenty of time for a deep dive into the actual IP of it all.
My main focus today is elsewhere: on what the backdrop of the suit says about the fundamental cost structure of LLMs and other generative content algorithms.
The Costs are Too Damn Fixed
OpenAI has already cut licensing deals with Axel Springer (Business Insider, Politico), and was in the process of negotiating one with the New York Times itself. This means they’ve fundamentally given up on the idea that what they’re doing is transformative enough to be considered “fair use,” even though the kind of guys who catch bullets for tech moguls online for free are still leaning on that canard.
And the fact that negotiations with the Times failed suggests, by the simplest inference, that the Times decided whatever amount of money OpenAI was offering wasn’t worth the anticipated cannibalization. In the meantime, OpenAI *already used* a ton of Times data without getting permission first. That opens them up for what could be a truly debilitating financial penalty, possibly in the multiple billions of dollars.
(Notably, the Times is represented by Susman Godfrey, the legal team that got an $800m punitive libel judgment against Fox News. I doubt they get out of bed in the morning unless they smell a reasonable chance of a huge payday.)
In this sense, OpenAI is basically Uber. But instead of trying to use legal arbitrage to get around local taxi licensing, they’re trying to arbitrage their way around the U.S. Constitution.
This adds to the evidence for a very simple argument: automated content generation has limited profit potential, and high fixed costs. Completely leaving aside concerns about the ethics of its training or its possible corrosive impacts on society, there is a strong case that LLMs and image generators fundamentally cannot work as sustainable businesses at a scale that justifies algorithm companies’ massive current implied enterprise value.
(Yes, they’re just *algorithms,* not “artificial intelligence” in any meaningful sense, and I try to avoid using that term, because it plays into the deceptive and dangerous legal and ideological agenda the industry is pushing.)
This in turn points to the larger deception fundamental to current AI operations. If ripping off content is implicitly unprofitable without free access to training data, these startups have to dangle something bigger - MUCH bigger - to attract funding. That bigger thing is “artificial general intelligence,” and there is no actual certainty this can ever be achieved. That’s why the “A.I. Doomers,” in their role of secret hype-men for the AI startups, must so loudly predict their terrible power, and even their sentience.
Anyway, if content automators have to pay licensing fees for their training data, they will likely have to do so in perpetuity – LLMs, for instance, would need constantly updated training data to remain relevant for a variety of hypothetical tasks. But added to the high cost of compute for these tasks, which isn’t coming down fast enough to disappear any time soon, this means automated content generation is fundamentally different, as a business proposition, from what Silicon Valley is used to.
The entire digital revolution was premised, for decades, on the idea of zero marginal cost. One fairly definitive book on the topic is Jeremy Rifkin’s. The basic idea is that software is infinitely copyable, so each additional unit you sell is in some sense pure profit.
This also applies to network tools like Facebook, and it’s fundamental to the way the tech economy works. Tech companies are able to “scale,” with few employees and huge profit margins, because of this infinite horizon of zero marginal cost.
But content generation algorithms have multiple marginal costs.
Keep reading with a 7-day free trial
Subscribe to Dark Markets to keep reading this post and get 7 days of free access to the full post archives.